Developing Hybrid-Based Recommender System with Naïve Bayes Optimization to Increase Prediction Efficiency
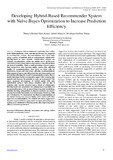
View/ Open
Date
2021-03Author
Ndung'u, Rachel N.
Kamau, Gabriel N.
Wambugu, Geoffrey M.
Metadata
Show full item recordAbstract
Commerce and entertainment world today have shifted to the digital platforms where customer preferences are suggested by recommender systems. Recommendations have been made using a variety of methods such as content-based, collaborative filtering-based or their hybrids. Collaborative systems are common recommenders, which use similar users’ preferences. They however have issues such as data sparsity, cold start problem and lack of scalability. When a small percentage of users express their preferences, data becomes highly sparse, thus affecting quality of recommendations. New users or items with no preferences, forms cold start issues affecting recommendations. High amount of sparse data affects how the user-item matrices are formed thus affecting the overall recommendation results. How to handle data input in the recommender engine while reducing data sparsity and increase its potential to scale up is proposed. This paper proposed development of hybrid model with data optimization using a Naïve Bayes classifier, with an aim of reducing data sparsity problem and a blend of collaborative filtering model and association rule mining-based ensembles, for recommending items with an aim of improving their predictions. Machine learning using python on Jupyter notebook was used to develop the hybrid. The models were tested using MovieLens 100k and 1M datasets. We demonstrate the final recommendations of the hybrid having new top ten highly rated movies with 68% approved recommendations. We confirm new items suggested to the active user(s) while less sparse data was input and an improved scaling up of collaborative filtering model, thus improving model efficacy and better predictions.
URI
https://www.ijcit.com/index.php/ijcit/article/view/75https://www.researchgate.net/publication/350499124_Developing_Hybrid-Based_Recommender_System_with_Naive_Bayes_Optimization_to_Increase_Prediction_Efficiency
https://www.semanticscholar.org/paper/Developing-Hybrid-Based-Recommender-System-with-to-Njeri-Ndung%27u/dfb688a226fa918ab822dd025f4d2c36741fd341
http://hdl.handle.net/123456789/6105
https://doi.org/10.24203/ijcit.v10i2.75
Collections
- Journal Articles (CI) [118]